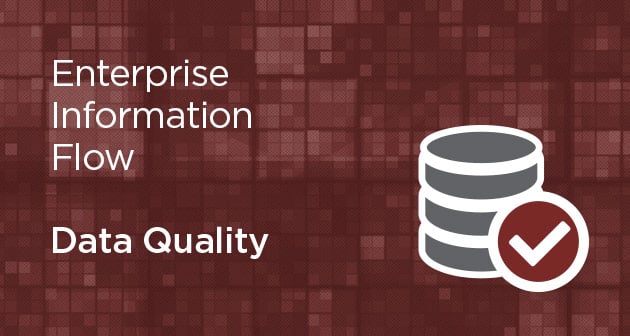
Data quality and the consequential quality of information are key issues in any organization dealing with big data flows. Taking data quality seriously is a necessity, especially since it is already a part of standard regulatory requirements. Let us illustrate the need for data quality assurance and its integration into your company’s Enterprise Information Flow using a simple case of Solvency II. Solvency II is a European Union directive for insurance companies, primarily made to prevent insolvency, but it’s also focused on data quality management issues. And what about the fact that your company might be based in the United States or any other part of the world? 1) Solvency II concerns some U.S.-based insurance companies too. 2) There is nothing wrong with getting your data quality processes straight. Your company can only benefit from it.
Requirements of Solvency II
Article 82 – Data quality and application of approximations, including case-by-case approaches, for technical provisions Member States shall ensure that insurance and reinsurance undertakings have internal processes and procedures in place to ensure the appropriateness, completeness and accuracy of the data used in the calculation of their technical provisions. Where, in specific circumstances, insurance and reinsurance undertakings have insufficient data of appropriate quality to apply a reliable actuarial method to a set or subset of their insurance and reinsurance obligations, or amounts recoverable from reinsurance contracts and special purpose vehicles, appropriate approximations, including case-by-case approaches, may be used in the calculation of the best estimate.
That does not sound too bad, right? Those requirements are widely specified in relevant documents. Basically, you need to know/document/be able to prove:
- all data used for calculating these required models and reports (business and technical descriptions)
- quality of all data used for these calculations and also intermediate results (on technological, functional, business and other levels)
- descriptions of all transformations and calculations used
- all places where data is being processed and where people interact with it
- people responsible for individual data sets and their processing
- data quality processes
- data quality measurements and reporting processes
- correctional processes and documentation of correctional processes
- other factors influencing reported information
This shows the necessity of connecting the employees who create and consume data, and also of educating them to be ready for errors. It also presents the necessity of using identical and exact metadata on both technological and business levels. Data quality has been employed very poorly in many companies, but theoretical research has been done properly. All the way back in 2002 Leo Pippino, Yang Lee and Richard Wang noted sixteen dimensions of data quality issues [pdf]:
- Accessibility – the extent to which data is available, or easily and quickly retrievable
- Appropriate Amount of Data – the extent to which the volume of data is appropriate for the task at hand
- Believability – the extent of which data is regarded as true and credible
- Completeness – the extent to which data is not missing and is of sufficient breadth and depth for the task at hand
- Concise Representation – the extent to which data is presented in the same format
- Ease of manipulation – the extent to which data is easy to manipulate and apply to different tasks
- Free-of-Error – the extent to which data is in correct and reliable
- Interpretability – the extent to which data is in appropriate languages, symbols, and units, and the definitions are clear
- Objectivity – the extent to which data is unbiased, unprejudiced, and impartial
- Relevancy – the extent to which data is applicable and helpful for the task at hand
- Security – the extent to which access to data is restricted approproately to maintain its security
- Timeliness – the extent to which the data is sufficiently up-to-date for the task at hand
- Understandability – the extent to which data is easily comprehended
- Value-Added – the extent to which data is beneficial and provides advantages from its use
There are also other classification procedures of issues with data quality, most notably with logical levels of use. Problems connected with low-quality data usage in different parts of organizations are listed in following table: Our experience shows that system fails are usually attributed to badly-set processes or poorly developed apps, but the problem is in fact low-quality data or information. That’s why it is necessary to use a new approach which will connect all logical levels with terminologies and techniques used for data quality requirements and their measurements in the data lifecycle. If you want to know more about Enterprise Information Flow, please take a look at our previous article about the benefits of Enterprise Information Flow or the introductory article EIF on our blog. Also, feel free to check us out on Twitter or get in touch via email.