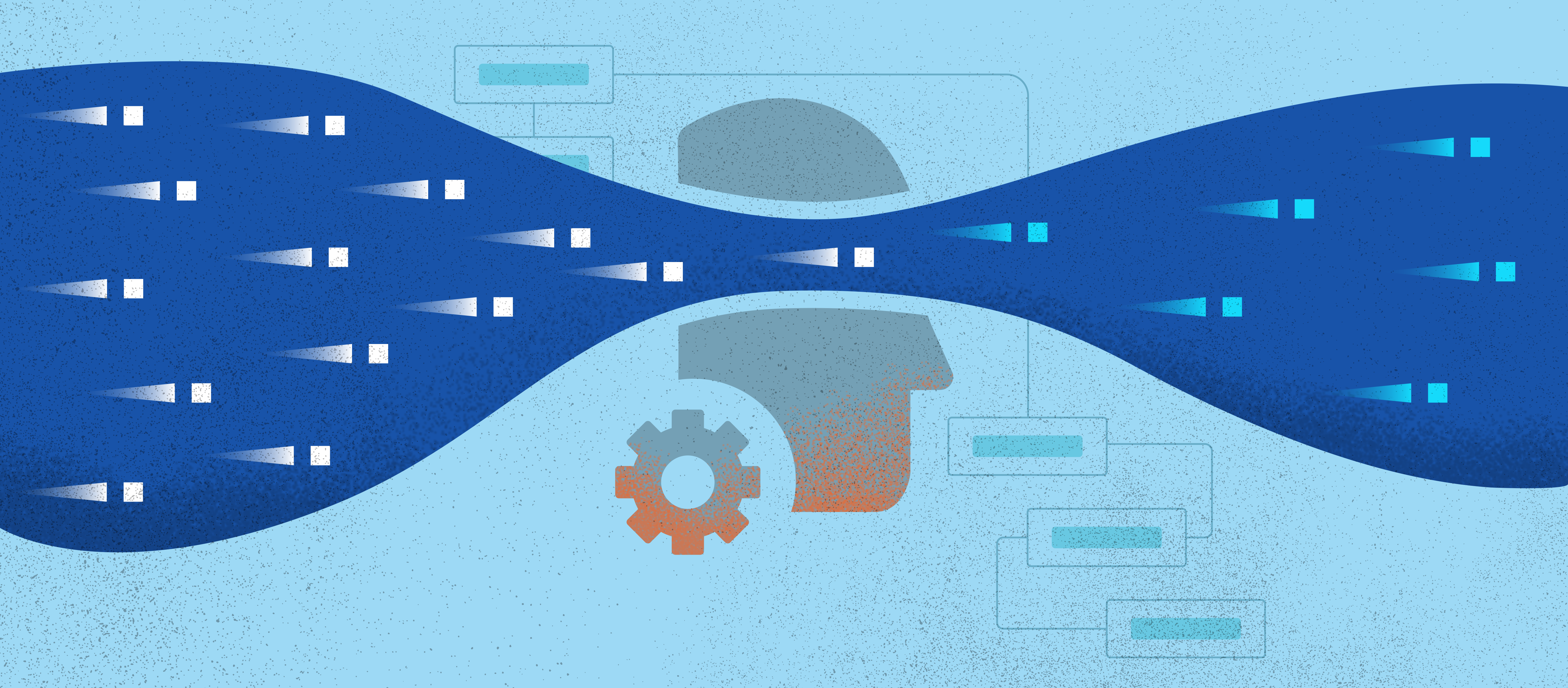
Have you heard of AI hallucinations? This phenomenon occurs when AI creates responses seemingly from nothing (or from completely incorrect data) and it can have huge costs. For example, a judge recently fined two lawyers and their law firm after they used ChatGPT to draft trial documents that included fake case law. In other instances, people have used generative AI to incorrectly self-diagnose health issues, leading to problems with people seeking treatment for diseases they don’t actually have.
So what can you do to prevent AI hallucinations? The answer lies in data lineage.
Understanding AI Hallucinations
The dangers of AI “hallucinations” — confident responses from an AI-powered chatbot that aren’t supported by its training data — extend into nearly every industry. In many instances, these hallucinations consist of responses that are an amalgamation of false-but-probable information from made-up sources.
When you consider the nature of the technology, it’s not astonishing that these hallucinations occur. We ask the technology to create works of fiction all the time, but then also ask it to create factual information – without the ability to discern between the two, the algorithm can’t keep up. But beyond that, if we don’t have the correct information fed into the technology to start, the hallucinations will only get worse.
Risks of AI Hallucinations
Depending on your industry, serious consequences can result from AI hallucinations, which goes beyond ChatGPT. For example, a wellness chatbot meant to replace a human-staffed helpline for people with eating disorders was suspended and is under investigation after it offered harmful advice to users.
The problem with AI models are, quite simply, that they are meant to make up answers. Thus, the incorrect information they produce — from risk modeling analytics to fraud analytics and predictive services — can mislead executives and business leaders about their operations. These false insights hamper asset management, damaging a business’s reputation and bottom line and jeopardizing jobs and operations in the process.
Generative AI depends on the data it's been trained with to build its ideas. It needs high-quality data to form high-quality information. But inherently, the nature of the algorithm is to produce output based on probabilities and statistics — not a true understanding of the content. The fact is this: Generative AI models are always hallucinating.
Now, let’s think about this in the context of a giant circle. The information being fed in is incorrect, the information being generated is used (confidently) by businesses and websites, which then feeds back into the AI… it is almost like playing a game of telephone, except instead of the end-result being a silly rumor that changed with every iteration, it is important business information.
While some experts in the field say they don’t fully understand how AI hallucinations emerge — or how to stop them — I believe we do understand their origins. But as I’ve said, we accept certain hallucinations that work in our favor based on the training sets and our criteria for the model’s output. The issue at hand is how to stop less favorable outputs in case users want to apply LLMs and other generative AI models to elicit more rigorous — and consequential — responses.
Introduction to Data Lineage
Traditionally, data lineage has been seen as a way of understanding how your data flows through all your processing systems—where the data comes from, where it’s flowing to, and what happens to it along the way.
In reality, data lineage is so much more. Data lineage represents a detailed map of all direct and indirect dependencies between the data entities in your environment. Why is this so important? Because a detailed map of your data can tell you if any of your data is incomplete, missing, in compliance with local laws, and who has access to it (as well as who changed it, and when). If this data is being fed into generative AI, lineage gives you the chance to see what data might be used and what data might have caused a hallucination in your internal generative AI. It might not 100% prevent hallucinations, but it can give you a fighting chance to get ahead of it.
The Role of Data Lineage in AI Hallucination Prevention
True generative AI, beyond LLMs like ChatGPT, depends on intricate data algorithms that encounter data obstacles like any other program. This complexity, combined with AI’s increasing adoption across fields, makes the need for auditable systems urgent.
Generative AI’s success hinges upon high-quality data. As mentioned earlier, data lineage enables organizations to root out harmful data to ensure data accuracy and quality. Developers can use automated lineage tools to trace the origins of training data. They can identify instances where the algorithm shifts sources to inaccurate information, whether that “bad” data flows from the wrong source or has been filtered in a way that introduces bias. This transparency is of utmost importance as generative AI faces stricter regulatory oversight and end users demand higher trustworthiness from AI tools.
Our Thoughts on the Future of AI Hallucinations
Although generative AI chatbots, like ChatGPT are still at the height of the hype cycle, these technologies are just the beginning for what AI can be truly capable of. That’s why the data being analyzed by the algorithms is so important.
Manta delivers transparency to the AI/ML “black box”, which helps our customer make their AI and machine learning pipelines “audit ready,” including
- Historical tracking for machine learning data sets over time.
- Tracking and identification of schema changes for learning data sets as well as when columns are changed, added, or deleted.
- Monitoring changes in transformations in data pipelines leading to generation of learning data sets.
Schedule some time to chat with our team to learn more about how Manta can help your AI initiatives. You’ll be glad you did.